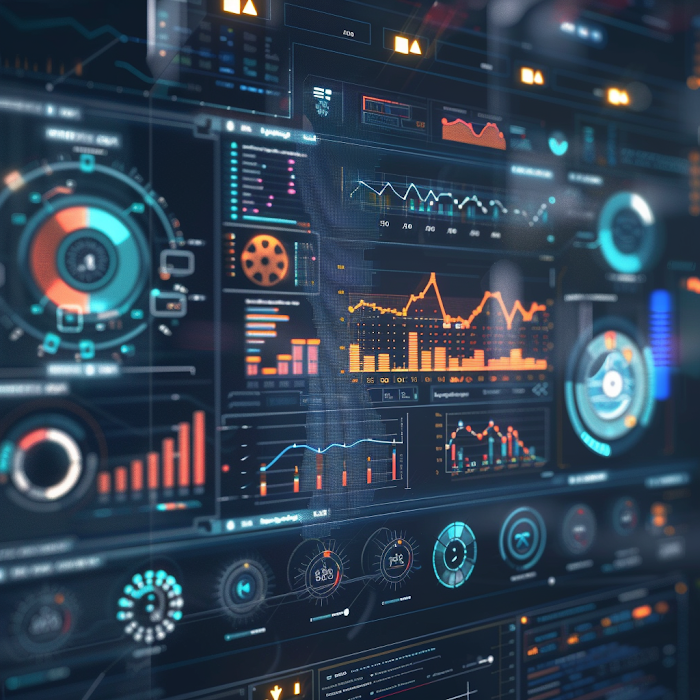
Unlocking Growth: The Power of Predictive Marketing
In today's marketing, leveraging big data through predictive analytics is essential for competitiveness. This involves using machine learning and AI to forecast trends, optimize campaigns, and predict consumer behavior. Benefits include personalized marketing, enhanced efficiency, and proactive strategies, supported by high-quality data and real-time analysis.
In today's rapidly evolving marketing landscape, the ability to effectively leverage big data for predictive marketing has become increasingly important for businesses wanting to outperform the competition. This data-driven strategy utilizes vast amounts of information alongside advanced analytical techniques to forecast marketing trends, optimize campaign effectiveness, and predict consumer behavior. By embracing predictive marketing, businesses can personalize customer experiences, streamline marketing efforts, and ultimately drive growth.
Understanding Predictive Analytics
Predictive analytics involves using historical data, machine learning, and AI algorithms to predict future outcomes. Over the years, predictive models have advanced from basic analytical frameworks to complex systems capable of analyzing enormous data sets with improved accuracy. This transition is driven by technological advances, including machine learning and artificial intelligence, which ensure that predictive models continuously evolve and learn from new data.
Key components of these predictive models include data quality, machine learning algorithms, and feature selection. High-quality data is vital to ensure accurate predictions, and it often involves integrating information from website interactions, social media, customer feedback, and other external variables. Machine learning algorithms such as decision trees and neural networks are critical for identifying patterns and forecasting future trends, while feature selection ensures the model selects the most impactful variables to avoid overfitting.
Applications in Marketing
One of the most potent applications of predictive analytics in marketing is in targeting decisions. Predictive models, especially logistic regression, enable marketers to predict the purchasing probabilities of consumers, optimizing which segments to target and thereby minimizing costs. This targeted approach enhances campaign productivity and ensures marketing resources are efficiently used.
Predictive marketing also shines in customer segmentation and personalized marketing. With the help of predictive models, businesses can segment customers based on shared traits and tailor marketing strategies to cater to specific segments. This allows for not only targeted marketing but also personalized recommendations, increasing engagement and conversion rates.
Furthermore, predictive models can identify patterns related to customer churn, allowing businesses to intervene before customers leave. By analyzing historical behavior, companies can undertake actions like offering promotions to retain at-risk customers. Sentiment analysis, another application, evaluates social media interactions and reviews to discern customer sentiment, helping businesses adapt their strategies based on consumer perceptions.
Increasing Business Efficiency
Big data in predictive marketing also enhances business efficiency by automating decision-making processes. Organizations can deploy scalable, cloud-based analytics platforms and AI solutions to process vast datasets, recognizing complex patterns that aid in accurate predictions. These insights can inform pricing strategies, cross and up-selling opportunities, or even real-time market response strategies.
Pioneering companies such as Amazon and Marriott demonstrate the incredible potential of predictive analytics. Amazon leverages big data to fine-tune customer experiences, optimize operations, and adjust pricing dynamically, whereas Marriott uses analytics to forecast demand precisely, monitor competitors, and tailor services to customer needs.
Best Practices and Advancements
To capitalize on predictive analytics, businesses must implement best practices to ensure their models are stable and not prone to overfitting. This involves establishing criteria for prediction accuracy assessments and employing methods like cross-validation. Moreover, AI greatly enhances predictive analytics through continuous data pattern learning, offering insights that prescribe future optimal decisions.
The importance of real-time data analysis and scalability cannot be understated. Big data analytics platforms capable of real-time processing allow businesses to gain immediate insights, enabling swift strategic responses to market dynamics. Leveraging AI and real-time analytics can set proactive marketing actions based on up-to-date trends.
In the end, data quality remains the cornerstone of effective predictive marketing. Businesses need to focus on gathering and integrating high-quality data from diverse sources to ensure the robustness of predictive models and the reliability of their forecasts.
Actionable Takeaways for Marketers
- Utilize predictive models for effective customer segmentation and personalized marketing campaigns.
- Implement churn prediction models to identify and retain at-risk customers proactively.
- Leverage sentiment analysis tools to monitor customer feelings and adapt strategies accordingly.
- Optimize pricing and marketing strategies using predictive insights to enhance consumer engagement.
- Automate marketing decisions by employing big data analytics tools for precise and timely predictions.
In conclusion, big data, when used effectively for predictive marketing, offers businesses a competitive edge by enhancing targeted marketing efforts and ensuring superior consumer engagement. By embracing advanced analytical techniques augmented by machine learning and AI, companies can accurately forecast marketing outcomes, personalize customer interactions, and achieve operational excellence.
#BigData #PredictiveMarketing #DataAnalytics #CustomerEngagement #AIinMarketing